Background
In daily conversations, people acquire information through auditory cues, such as voice, and visual cues, like lip movements, to facilitate the comprehension of spoken content. The audio may be drowned in the noise encountered in poor acoustic scenarios, making the content difficult to acquire. Lipreading infers content through lip movements, lying at the intersection of computer vision and natural language processing. In public security, lipreading is crucial in detecting facial forgery and determining liveness. Moreover, advances in lip reading also drive the development of audio-visual speech recognition and enhancement, providing an effective way to input video as a complement in challenging acoustic environments.
Currently, lipreading tasks primarily concentrate on English, emphasizing the crucial need for increased attention in Chinese. The heightened complexity of Chinese lipreading stems from the extensive number of Chinese characters and their complex relationship with lip movements. Additionally, the lack of large-scale Chinese datasets constrains research efforts. The existing open-source Chinese lipreading datasets are mostly from controlled settings like laboratory recordings. The contents of these videos are carefully prepared, leading to a more presentational style rather than colloquial chatting. Figure 1 illustrates examples of current open-source lipreading datasets and ours (MISP).
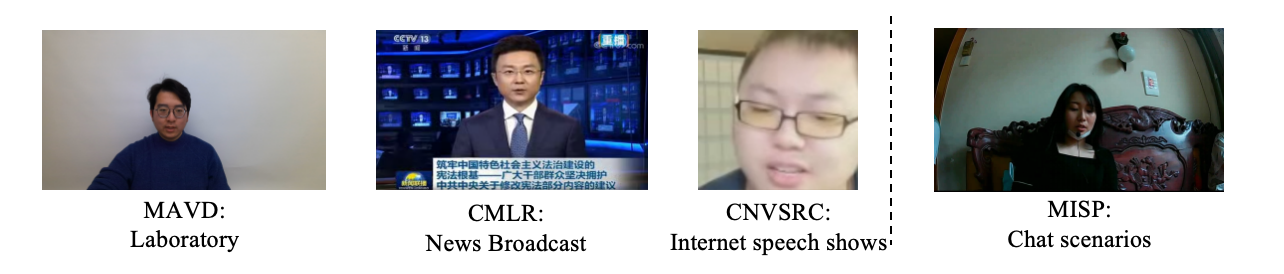
Lipreading can be categorized into two subtypes: word-level and sentence-level. The research on word-level lipreading has been extensively conducted. Sentence-level Chinese lipreading is even more challenging. Due to the inherent ambiguity in lip shapes, different words may exhibit similar lip movements. Variations in articulation habits might lead to different lip movements for the same word. The accuracy of sentence-level recognition is too low for practical use. Therefore, we narrow the focus of general sentence-level lipreading system to speaker-specific taks.
Considering the above perspectives, we organize the ChatCLP challenge in real home chatting environments to address wake word and target speaker lipreading. We are dedicated to fulfilling the requirements of waking up smart home devices within household settings and utilizing video for speech recognition with these smart home devices.